It’s a conscious choice between outdated, largely manual curb management or smarter, informed decision-making.
While the curb has traditionally been an overlooked resource in urban planning, its value continues to increase exponentially. Faced with a myriad of competitive stakeholder demands and pressure, cities must shift and embrace data science to transform how we use, monitor, and regulate this essential space.
Data science isn’t just about delivering reports and statistics. It’s a multidisciplinary field combining scientific methods, algorithms, and programming to analyze and interpret vast amounts of data. By uncovering patterns, trends, and relationships in both structured and unstructured data, data science delivers insights enabling cities to make to data-driven decisions that unlock the full power of the curb.
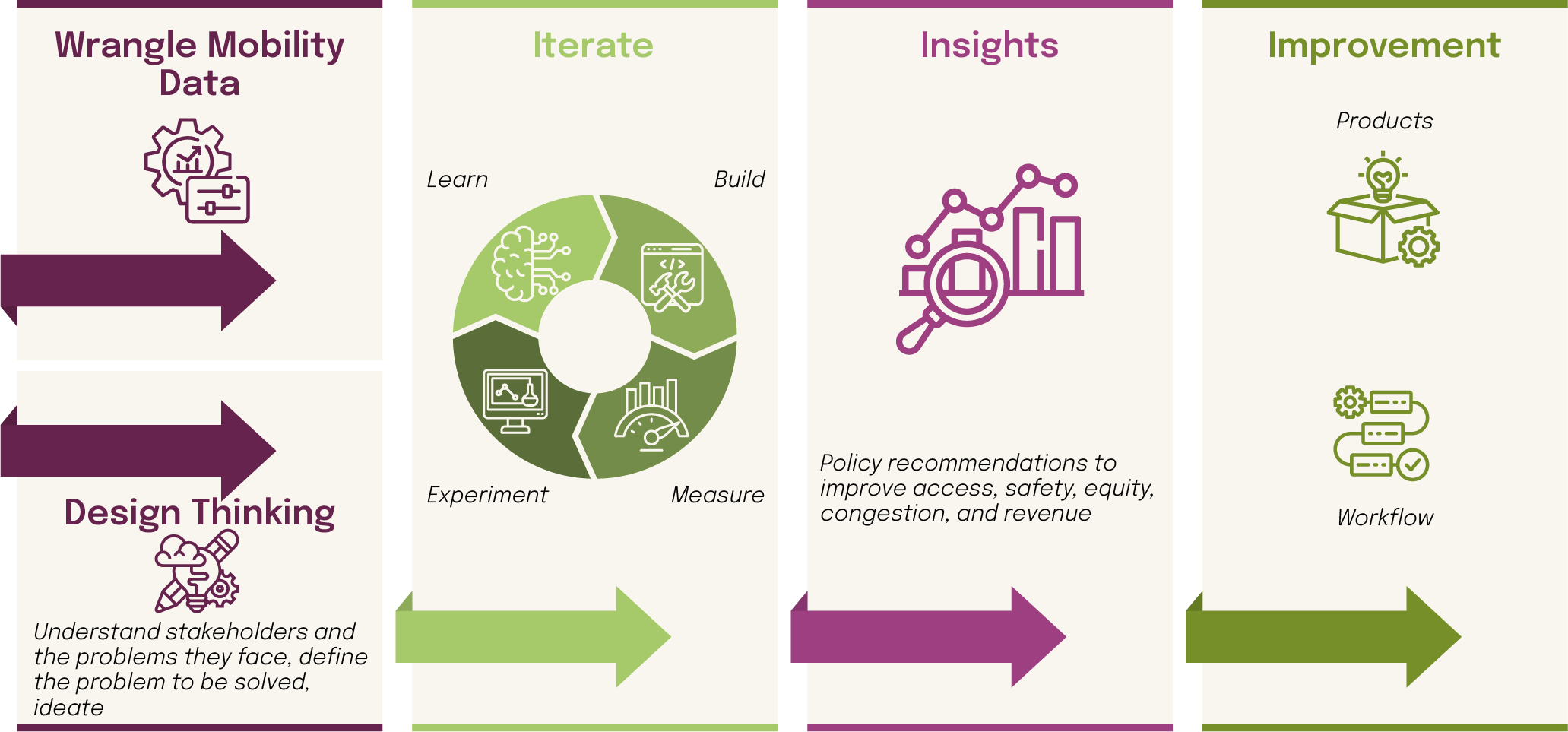
Collecting data. Data wrangling is the foundational step in the data science process. It involves gathering raw data from various sources—like meters, sensors, parking complaints, and citation issuance—as well as heterogeneous data like accidents, census tract demographic and socio-economic information, and street miles and types—cleansing it by handling missing values, correcting errors, and transforming it into a structured format.
Cities need not deploy a sensor or camera covering every space in real-time. There are sustainable data collection methods, including temporal and spatial sampling, that can paint a picture of curb use with a high degree of confidence and accuracy.
Design Thinking. In addition to data, we need to frame curbside problems and understand the needs of stakeholders, defining the key problems to address and ensuring that data-driven solutions align with the broader business objectives. By emphasizing empathy and creative problem-solving, design thinking helps create solutions that are both practical and impactful.
Iterate—Experiment, Learn, Build, Measure. The iterative phase is where data science truly shines. It involves continuously refining models and hypotheses based on feedback, experimentation, and measurement. Data scientists learn from each iteration, adjusting models to improve accuracy and performance. Experimentation is crucial here, as it allows for testing different approaches, fine-tuning algorithms, and optimizing solutions before final implementation.
Deliver Insights. Unlike data analytics, data science doesn’t push the work of developing actionable curbside insights to already over-burdened city officials. Rather, once models are developed and refined, data scientists present results in a clear, digestible format, often supported by visualizations. The goal is to make complex data understandable, highlighting trends, patterns, and predictions that can drive parking strategies and improvements.
Embed in Products, Workflow. The final step is integrating the insights and models into business processes or products for continuous use. This could mean embedding predictive models and machine learning into demand pricing engines, automating parking enforcement assignments, measuring occupancy with asset light data collection, or enhancing workflows with data-driven recommendations. By embedding data science into everyday parking operations, cities can ensure that data-driven insights are consistently leveraged to optimize performance and drive long-term success.
The big takeaway?
Data can transform curbside management from reactive to proactive. Innovative cities worldwide are already reaping the benefits. Here are five ways insights can transform your city:
Rather than mass mail notices of outstanding parking debt, the City of Oakland used data to prioritize notices to motorists most likely to pay. Doing so helped to reduce costs while optimizing critical end of year revenues.
The City of Los Angeles used machine learning models to predict demand fluctuations and adjust pricing, shifting motorists to underutilized areas and reducing congestion. Demand pricing increases hourly meter rates during peak hours and reduces rates during off-peak times, encouraging more efficient use of valuable curb space.
Sustainable, “asset light” data collection can help cities code the curb, understand demand, and improve loading zone policies. Cities like Columbus are using data to modify the operable hours of loading zones, change the size of those zones, and shift zones to different locations to optimize the use of the curb.
And in Chicago, coding the curb is helping the City enforce bike and bus lanes with vehicle-mounted cameras. Automated enforcement is premised on accurate curbside data, and we’ve worked with the City to identify curbside assets to improve safety. Further, we’re working to better allocate enforcement vehicles to optimize routes.
In Washington, DC, properly pricing parking meters cut stays, reduced circling for a parking space, and shifted demand. Demand pricing effectively reduced CO2 while simplifying the parking experience.
- Make parking enforcement more equitable
Disadvantaged motorists often bear a disproportionate brunt of enforcement. But data driven insights can improve the allocation of enforcement, alleviating that burden. Chicago’s work to improve the deployment of parking enforcement officers has reduced the percentage of tickets in disadvantaged communities as well as the average fine values. Further, data can help inform optimized parking fines, equitable hearing policies, and scofflaw enforcement strategies.
As we move towards smarter cities, embracing data science for curb management is not just a luxury; it’s a necessity. With better insights and data-driven strategies, cities can unlock efficiencies, reduce congestion, and enhance the urban experience for everyone. It’s time to rethink the curb—not as a fixed, static space, but as a dynamic resource that can be optimized for the needs of the modern city.